Our paper introduce two main ideas for tackling 4D Lidar Panpoptic Segmentation.
i) Forming 4D volumes using consecutive lidar frames using ego-motion and obtain instance and semantic segmentation predictions on both space and time.
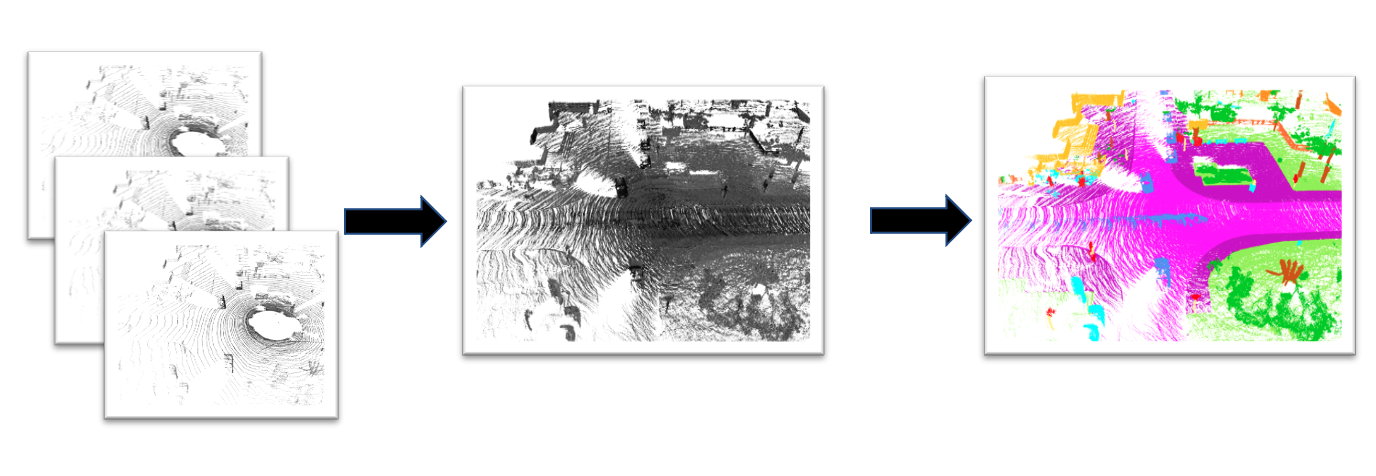
ii) A point-based encoder-decoder network which works on 4D volumes and outputs necessary ingredients to cluster points via gaussian distribution function, as well as semantic interpretation for all points.